Word frequency analysis
- The Words They Used (NY Times visualization of the 2008 US political conventions)
- Wordle
- R. Luke Dubois, Hindsight is always 20/20
Chance operations
We’ll discuss here several varieties of “non-intential” composition (which may or may not incorporate “chance” in the sense of “randomness.”) These categories overlap a great deal, and there’s obviously a lack of clarity about where to draw the lines between them. Which of these can be reproduced algorithmically?
Aleatory. “Some element of the composition is left to chance, and/or some primary element of a composed work’s realization is left to the determination of its performer(s). The term is most often associated with procedures in which the chance element involves a relatively limited number of possibilities.” (from Wikipedia. Examples: Automatic writing, John Cage’s early mesostics.
asK Little autO Where it wantS To take You.
Deterministic. A non-intentional process that leads to the same result every time. (No chance or choice involved.) Examples: Jackson Mac Low’s diastics and asymmetries. Asymmetry 205, There are many ways to use Strayer’s Vegetable Soybeans:
To hours, enough. Remove enough
And. Remove enough
Minutes. And not Iowa
Water and Iowa simmer.
To or
Until simmer. Enough
Simmer. To. Remove and Iowa enough. Remove simmer.
Vegetable. Enough good enough to and buttered loaf, enough
Simmer. Or Iowa buttered enough and not simmer.Tomatoes, hot egg. Roll egg.
Added. Roll egg.
Minutes. Added, nutty in.
Wash added, in soak
Tomatoes, overnight,
Until soak egg,
Soak tomatoes. Roll added, in egg. Roll soak
Vitamins—egg, giving egg, tomatoes, added, beans, largest egg,
Soak overnight, in beans, egg, added, nutty soak
Stochastic. Existing parts are re-arranged and juxtaposed using chance. Example: Raymond Queneau’s Cent mille milliards de poèmes.
Random numbers in Python
Python makes it easy to work with random numbers. The random module includes several functions for generating random numbers and choosing random items from lists. Here’s a sample transcript from the interactive interpreter:
>>> import random >>> random.random() # random number between 0 and 1 0.90685046351757992 >>> random.randrange(1, 10) # random number from 1 to 10 2 >>> random.gauss(0, 1) # gaussian random, mean 0, stddev 1 -0.15235026257945011
Python: Simple models of text
So far, we’ve been working with programs that examined just one line of a file at a time. During this session, we’ll be expanding our scope a little bit: we want to make programs that can build a picture of how an entire text looks, seems and behaves. In order to facilitate that, we’ll be looking at a few simple data structures.
Lists
Lists in Python are a kind of object that stores other objects. (They’re a lot like arrays in Processing, but more powerful, as you’ll see.) Once you’ve created a list, or put objects into a list, you can retrieve them using the same syntax we used last week to get individual characters out of strings. You can also get slices of a list, using the same syntax we used to get slices of strings. Here’s some example code:
>>> parts = ['led', 'resistor', 'capacitor'] >>> len(parts) # how many elements are in the list? 3 >>> type(parts) # what kind of object is this? <type 'list'> >>> parts[1] 'resistor' >>> parts.append('ultrasonic range finder') # adds a new element to list >>> parts ['led', 'resistor', 'capacitor', 'ultrasonic range finder'] >>> parts[2:] ['capacitor', 'ultrasonic range finder'] >>> parts.sort() # sorts the list in-place (i.e., changes the list) >>> parts ['capacitor', 'led', 'resistor', 'ultrasonic range finder'] >>> parts.reverse() # reverses the list in-place >>> parts ['ultrasonic range finder', 'resistor', 'led', 'capacitor'] >>> 'led' in parts # is the string 'led' in the list? True >>> 'flex sensor' in parts False >>> more_parts = [] # create an empty list >>> more_parts = list() # same thing
As you can see, list literals are made by surrounding a comma-separated list of objects with square brackets. You can store any kind of object in a list: strings, integers, floats, even other lists (or sets or dictionaries)!
You can iterate over the elements of a list with for, just like you iterate over the individual characters of a string. Here’s a transcript to demonstrate, from the interactive interpreter:
>>> materials = ['poplar', '8-segment LED', 'photoresistor', 'felt', 'lard'] >>> for material in materials: ... print material ... poplar 8-segment LED photoresistor felt lard
What if I just want to count from one to ten?
Use Python’s built-in range() function, which returns a list containing numbers in the desired range:
>>> range(1,11) [1, 2, 3, 4, 5, 6, 7, 8, 9, 10]
This script:
for i in range(1,6): print i
Will output:
1 2 3 4 5
Randomness with lists
Python’s random library provides two helpful functions for performing chance operations on lists. The first is shuffle, which takes a list and randomly shuffles its contents; the second is choice, which returns a random element from the list.
>>> import random >>> cards = ['two of cups', 'four of swords', 'the empress', 'the fool'] >>> random.shuffle(cards) >>> cards ['two of cups', 'the fool', 'four of swords', 'the empress'] >>> random.choice(cards) 'the fool'
Data structures to store text: randomize_lines.py
This brings us to our first full-fledged example program, randomize_lines.py. Instead of operating on one line at a time, this program stores all of the lines from standard input into a list, then uses the random.shuffle
function to print out the lines in random order. Here’s the code:
import sys import random all_lines = list() for line in sys.stdin: line = line.strip() all_lines.append(line) random.shuffle(all_lines) for line in all_lines: print line
The all_lines
variable points to a list. Inside the first for
loop, we add each line that comes in from standard input to the list. After calling shuffle
to re-order the list, we then print the list back out. If you pass in Sea Rose, you’ll get back a delightful re-imagining:
spare of leaf, hardened in a leaf? drip such acrid fragrance in the crisp sand Stunted, with small leaf, Rose, harsh rose, single on a stem -- Can the spice-rose that drives in the wind. than a wet rose you are caught in the drift. you are lifted meagre flower, thin, you are flung on the sand, marred and with stint of petals, more precious
Strings and lists: split and join
String objects in Python provide two helpful functions to break strings up into lists of strings, and join lists of strings back into a single string. The split method “splits” a string into a list of strings, using the parameter that you pass to the method as a delimiter. The join method uses whatever string you call it on to join together the list of strings passed in as a parameter, creating a list. These are a little tricky, so it’s helpful to see them in action. Here’s a transcript from the interactive interpreter:
>>> foo = "mother said there'd be days like these" >>> foo.split(" ") # split on white space ['mother', 'said', "there'd", 'be', 'days', 'like', 'these'] >>> foo.split("e") # split on the letter "e" ['moth', 'r said th', 'r', "'d b", ' days lik', ' th', 's', ''] >>> wordlist = ['this', 'is', 'a', 'test'] >>> separator = " " >>> separator.join(wordlist) 'this is a test' >>> " ".join(wordlist) # same thing 'this is a test'
We’ll most often be using the split method as a shorthand for “split this string into a list of words.” (We’ll find more robust solutions for the problem of parsing words from a string when we discuss regular expressions.) Here’s a program that shuffles the order of the words on each line of standard input (available in the examples as randomize_words.py):
import sys import random for line in sys.stdin: line = line.strip() words = line.split(" ") random.shuffle(words) output = " ".join(words) print output
Here’s the result from passing in our favorite Robert Frost poem:
Two in yellow roads diverged a wood, could sorry I not And both travel traveler, be one stood I And long And I as could down one far looked as bent in undergrowth; To where the it other, fair, just took as Then as the better the perhaps having claim, And and it was grassy Because wear; wanted the that for passing there Though as worn them Had same, really the about both equally lay that morning And no trodden step had In leaves black. kept I day! the another Oh, for first way, knowing to Yet on leads way how come back. if doubted I should I ever be a this telling with I shall sigh hence: ages ages Somewhere and diverged I— wood, a and roads in Two less the one I travelled by, took all has difference. that And the made
sys.argv: Python’s important built-in list
Last week we learned how to run our Python scripts from the command line, as though they were UNIX text mungeing utilities. Most UNIX utilities take arguments on the command line: grep takes a pattern to search for, for example. We can read command-line parameters from Python as well, using the sys.argv list. This list contains all of the parameters passed on the command line, including the same of the script itself.
For example, take the following script, called argv_reader.py:
import sys for arg in sys.argv: print arg
If you ran it on the command line like so:
$ python argv_reader.py cat wallaby armadillo
You’d get the following output:
argv_reader.py cat wallaby armadillo
Sets
The set is our second important data structure. You can think of a set as a kind of list, but with the following caveats:
- Sets don’t maintain the order of objects after you’ve put them in.
- You can’t add an object to a set if it already has an identical object.
Objects can be added to a set by calling its add method (as opposed to the append method used for lists).
A corollary to #1 above is that you can’t use the square bracket notation to access a particular element in a set. Once you’ve added an object, the only operations you can do are to check to see if an object is in the set (with the in operator), and iterate over all objects in the set (with, for example, for). Here’s a transcript of an interactive interpreter session that demonstrates these basic features:
>>> foo = set() >>> foo.add(1) >>> foo.add(2) >>> foo.add(3) >>> foo set([1, 2, 3]) >>> foo.add(1) # will be ignored---only one of any identical object can be in set >>> foo set([1, 2, 3]) >>> 1 in foo True >>> 5 in foo False >>> for elem in foo: ... print elem ... 2 1 3
An additional aspect of sets to note from the transcript above: because sets don’t maintain the order of objects, you’ll get the objects back in (seemingly) random order when you iterate over the set. For most applications, this isn’t a problem, but it’s something to keep in mind.
Sets: the gritty details
So how does the set object work? How can it tell whether or not something has already been put into it? Here’s the basic gist, leaving aside some of the frothy complications. (See the Wikipedia page for a more detailed explanation.)
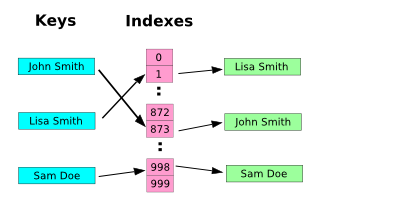
set diagram
(adapted from Wikipedia)
Every object that you put into a set is first sent through a hashing algorithm. The point of this algorithm is to generate a unique number (a “hash”) for every object that you pass to it. In the example above, the hashing algorithm returns the number 873 for string “John Smith”; the number 1 for the string “Lisa Smith”; and the number 998 for the string “Sam Doe.” These numbers are then used as an index to an array. When you call the add
method of a set object, whatever you passed to it gets stored in that array at the index returned by the hashing algorithm. When you ask the set (using the in
operator) if a particular object is present, it calculates the hash for that object, looks it up in the array, then returns True
if something’s there (and False
otherwise).
Now we can explain why the set doesn’t return objects in the same order they were put in: the set doesn’t have the means to remember the order. When you iterate over the set (using, e.g., a for
loop), it returns objects in the order of their hash—that’s the only order the set knows.
“But but but,” you say. “Couldn’t I do all this with a regular list?”
You could! It is, of course, possible to check a list for duplicates, and indeed the list class provides a means to do just such a thing—the in
operator. We can easily imagine what some code that implements the functionality of the in
operator might look like:
def list_contains(list_to_search, thing_to_search_for): for item in list_to_search: if item == thing_to_search_for: return True return False
The problem with this function is easy to spot: in order to see if thing_to_search_for
is in list_to_search
, we potentially have to look at every single element of list_to_search
. If we’re checking for a duplicate entries in a large list, this can slow a program down. (This is what’s called an O(n) operation: in order to complete the operation, you potentially need to perform n comparisons, where n is the length of the list.)
If we use a set, on the other hand, we only need to perform a single check to determine if an object is already present in the set: just look at the index associated with that object’s hash value. For large amounts of data, this is much, much faster. (This is called an O(1) operation: only one check is needed, regardless of how many items are in the set.)
Aside: Hashing algorithms
From a conceptual perspective, a hashing algorithm is a simple thing: we can think of all kinds of ways to turn (e.g.) a string into a number. We could add together the ordinal value of the characters in the string, for example (‘a’ = 1, ‘b’ = 2 …), or assign each word to its order in the dictionary (“aardvark” = 0, …, “zyzzyva” = 204,254).
It turns out, however, that designing a hashing algorithm suitable for storing data is fairly difficult. The ideal algorithm needs to return numbers that are unique for every input—even similar strings should return different numbers—and should provide values that are uniformly distributed over a given range. This is the kind of stuff that people get Computer Science degrees to understand (though the Wikipedia page on hashing algorithms is a good introduction). Thankfully, we don’t need to bother with the details, because Python’s taking care of us. All of the hash value calculations are abstracted away in the set class.
Applications of the set
Sets are great when you want to store data, but you want to ignore duplicates in the data. One classic example is to create a list of unique words in a text file. Here’s a program that does just that, available in this session’s example programs as unique_words.py:
import sys words = set() for line in sys.stdin: line = line.strip() line_words = line.split() for word in line_words: words.add(word) for word in words: print word
The important lines in this program are lines 8 and 9, in which we loop over every word from the current line and add them to the set. Because sets ignore any attempt to add in an object that is already in the set, once we’ve inserted one word, the set will only ever contain one copy of that word.
On lines 11 and 12, we loop over the contents of the set and print them out. (Note here again that the words in the set won’t appear in any particular order.) Here’s some sample output, obtained by running the program with sea_rose.txt as input:
and lifted flower, flung meagre single rose, in acrid stint harsh rose caught that leaf? drift. fragrance wet hardened thin, more wind. Stunted, leaf, sand, drives Rose, stem spare Can such precious with than a on crisp -- of drip petals, sand spice-rose you small the marred are
Dictionaries
The ”dictionary” is a very powerful data structure. You can think of it as an array whose indices are strings (or any other object) instead of numbers. In PHP, they’re known as ”associative arrays” and in Perl they’re ”hashes”; in Java, there’s a class called ”Map” that does the same thing. Dictionary literals in Python consist of comma-separated key/value pairs, with a colon between the key and the value (see the transcript below for an example). Keys can be any object (with some exceptions); values can be any object. You can access values of a dictionary with square brackets, much like the list indexing syntax. Some sample code for the interactive interpreter:
>>> assoc = {'butter': 'flies', 'cheese': 'wheel', 'milk': 'expensive'} >>> assoc['butter'] # access value at a key 'flies' >>> assoc['gelato'] = 'delicious' # assign to a key >>> assoc.keys() ['butter', 'cheese', 'milk', 'gelato'] >>> assoc.values() ['flies', 'wheel', 'expensive', 'delicious'] >>> 'milk' in assoc True >>> 'yogurt' in assoc False >>> foo = {} # create an empty dictionary >>> foo = dict() # same thing
You can think of a dictionary as a kind of set that contains not just a set of unique keys, but also a series of values associated with those keys. Schematically, it looks like this:
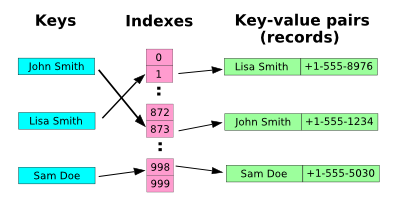
dictionary diagram
This diagram looks a lot like the set diagram above, but look closely—the dictionary stores not just the key, but a value associated with that key. The data structure is called a dictionary because you “look up” a key in order to get its “definition” (i.e., its value). In the case of our program that counts the number of times unique words occur in a text, strings (words) are the keys and integers (the number of times that word occurs) are the values.
The concordance
So what are dictionaries good for? One classic application is to build a simple concordance: a list of words that occur in a text, and how many times those words occur. Here’s the source listing for just such a program (concordance.py):
import sys words = dict() for line in sys.stdin: line = line.strip() line_words = line.split(" ") for word in line_words: if word in words: words[word] += 1 else: words[word] = 1 for word in words.keys(): print word + ": " + str(words[word])
This program illustrates several important idioms for working with dictionaries:
- It’s okay to assign to a key that doesn’t already exist in a dictionary, but it’s an error in Python to try to access a non-existent key. That’s what the code in lines 9-12 is for: first we check to see if the current word is already in the dictionary; if it is, then we increment its value by one. Otherwise, we assign a value to that key.
- There are many ways to iterate over the contents of a dictionary. One is to iterate over the list of keys returned from the dictionary’s keys method, then access the corresponding value by using that key as an index.
The dictionary data structure is one of the handiest data structures out there. It’s convenient, intuitive, fast, and (when implemented correctly) fairly memory-efficient. It’s a power tool for programming. Once you know how dictionaries work, you’ll probably find yourself figuring out all kinds of ways to apply the data structure to the problem at hand.
Dictionaries don’t just have to map string keys to integer values; they can map arbitrary objects to other arbitrary objects. We’ll be making programs that have lists as values, or other dictionaries as values, for example.
Reading data from a file
Up to this point, all of our programs have been operating on the standard UNIX input and output streams. Occasionally we will have the need to read from files other than (or in addition to) standard input. Fortunately, Python makes this easy: just use the open
built-in function.
Interactive transcript demonstrating open
and the object it returns:
>>> file_obj = open('wasteland.txt') # creates a file object from 'wasteland.txt' in the current dir >>> type(file_obj) <type 'file'> >>> dir(file_obj) # what methods do file objects support? ['__class__', '__delattr__', '__doc__', '__enter__', '__exit__', '__format__', '__getattribute__', '__hash__', '__init__', '__iter__', '__new__', '__reduce__', '__reduce_ex__', '__repr__', '__setattr__', '__sizeof__', '__str__', '__subclasshook__', 'close', 'closed', 'encoding', 'errors', 'fileno', 'flush', 'isatty', 'mode', 'name', 'newlines', 'next', 'read', 'readinto', 'readline', 'readlines', 'seek', 'softspace', 'tell', 'truncate', 'write', 'writelines', 'xreadlines'] >>> lines = file_obj.readlines() # readlines() returns an array of all the file's lines >>> len(lines) 476 >>> lines[0] 'I. THE BURIAL OF THE DEAD\n' >>> for line in open('wasteland.txt'): # or, we can use a for loop to loop over each line of the file ... print line ... # prints out every line from 'wasteland.txt'
List comprehensions
When you’re programming computers, there frequently arises the need to create a list that is a copy of another list, except with certain elements modified or filtered. Normally, you’d write code following this pattern:
source = variable of type list dest = list() for item in source: if condition: dest.append(expression)
Python has a special syntactic structure called a list comprehension to condense this logic into one line:
dest = [expression for item in source if condition]
Where dest is the new list, source is the source list, expression is some Python expression using item, and condition is a Python expression that evaluates to true or false. The new list will contain whatever expression returns for each item in the list, sequentially, if the condition for that item evaluates to true. (The if clause is optional; if omitted, no items from the source list are skipped.)
(If you’re familiar with SQL, you can think of a list comprehension as being analogous to a SELECT statement: SELECT expression FROM source WHERE condition.)
A quick interactive session to demonstrate:
>>> [x*x for x in range(10)] # squares of numbers from 0 to 9 [0, 1, 4, 9, 16, 25, 36, 49, 64, 81] >>> [x*x for x in range(10) if x % 2 == 0] # squares of only even numbers from 0 to 9 [0, 4, 16, 36, 64] >>> words = ['it', 'was', 'the', 'best', 'of', 'times'] >>> [w.upper() for w in words] # copy of list words, but in upper case ['IT', 'WAS', 'THE', 'BEST', 'OF', 'TIMES'] >>> [w for w in words if len(w) == 3] # only those words with a length of 3 ['was', 'the'] >>> [w[0].upper() for w in words] # just the first character of each item in words ['I', 'W', 'T', 'B', 'O', 'T']
The advantage of list comprehensions is that they more elegantly express our intent.
Putting it all together: adj_extract.py
Here’s an example program that demonstrates everything we’ve talked about in this week’s notes: adj_extract.py. This program prints out all adjectives present in the input. If you run the program passing in Sea Rose as input, you’ll get output that looks like this:
harsh meagre precious small acrid
How does the program work? Take a look at the source code, and I’ll walk you through it.
import sys adj_set = set() for line in open('adjectives'): line = line.strip() adj_set.add(line) for line in sys.stdin: line = line.strip() adjs = [s for s in line.split(" ") if s.lower() in adj_set] if len(adjs) > 0: print ', '.join(adjs)
As you can see, there are two loops that iterate over files in this program. The first iterates over the lines in a file called adjectives (included in the examples repository), and adds the content of each line to a set called adj_set. The adjectives file simply contains a list of adjectives in the English language, one per line; so when the loop is complete, adj_set will be a set containing all of the adjectives from the file.
Pop quiz! Why use a set here instead of a list? Consider how we’re using the data structure later in the program.
The second loop (beginning with for line in sys.stdin) loops over every line in standard input, splits that line up into words, and checks to see if any of the words are present in adj_set. Any matching words are printed to output, separated by commas.
The most confusing line here is the one containing the list comprehension, so let’s look at that line in more detail.
adjs = [s for s in line.split(" ") if s.lower() in adj_set]
What’s happening here, exactly? Let’s take it from the inside out.
for s in line.split(" ")
This is the central chunk of the list comprehension. This is saying: “We’re going to make a new list from the list returned from line.split(" "), and inside the list comprehension, each element of the list will be known (temporarily) as s.”
Expanding a little bit:
s for s in line.split(" ")
The s here at the beginning is telling Python what value we want to end up in our target list (adjs, in this case). The fact that this expression is exactly the same as the name of the temporary variable in the loop simply means that we want the exact value that came from the source list (line.split(" ")). (We could have used another expression—try replacing the above with s.upper() for s in line.split(" "), for example.)
s for s in line.split(" ") if s.lower() in adj_set
The if clause at the end here tells Python that we only want this element of the source list to end up in our target list if some particular expression holds true. In this case, the expression is s.lower() in adj_set—in other words: is the lower case version of this element of the list present in adj_set? If so, s will end up in the target list; if not, s will be skipped. (Why lower case? Because all of the words in the adjectives file are in lower case.)
adjs = [s for s in line.split(" ") if s.lower() in adj_set]
All together, now: the list comprehension (everything between the square brackets) evaluates to every element of the list returned from line.split(" "), if that element (transformed into lower case) is present in adj_set. Assign the result of the list comprehension to adjs.
Mini-exercise: Translate this list comprehension into a loop.
Exercises
We’ll do some of these in class.
1. How would you write a program that randomizes the words in each line of standard output, then prints out the randomized lines in random order? Can this be done without any writing any new code at all?
2. Let’s say that we wanted our concordance script to store not just how many times a particular word occurred, but on which lines. How would we go about doing that? What kind of data structure would we need? What additional information would we need that we aren’t already tracking in concordance.py?
3. The alpha_replace.py reads in a text file as a source file, then replaces the words in standard input with words in the source file that begin with the same letter. Write a version of this script that replaces words not according to their first letter, but according to the number of letters in the word.
4. Make any of the example scripts insensitive to case.
5. Re-write the following code to use a list comprehension.
names = ['Joe', 'Bob', 'Gerty', 'Ross', 'Andy', 'Mary'] mod_names = list() for n in names: mod_names.append("It's the " + n + "-meister!")
Further reading
- Lists from How to Think Like a Computer Scientist
- Dictionaries, Oh Lovely Dictionaries from Learn Python the Hard Way
- List comprehensions tutorial
Reply
You must be logged in to post a comment.
No comments
Comments feed for this article
Trackback link: http://www.decontextualize.com/teaching/rwet/simple-models-of-text/trackback/