The Glasgow Science Center is currently exhibiting The Joking Computer, a kiosk-based installation running software made by artificial intelligence researchers at the University of Aberdeen. The software uses phonetic information about English words and semantic information from WordNet to generate pun-based riddles. (More information about how it works.)
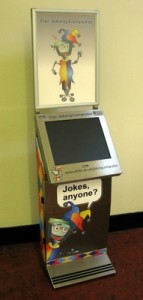
A few of my favorites:
What kind of tree is nauseated?
A sick-amore
What do you call a cross between an emporium and a success?
A department score
What do you get when you cross a choice with a meal?
A pick-nic
More examples here, and an online version is promised soon.
I like to read programs like this—programs that generate text conforming to a specific genre—as a kind of ethnographic criticism. The Joking Computer describes a particular type of joke by observing how jokes of that type are formed and used in our culture, and then formalizing the jokes as a procedure. The procedure itself serves as a statement about how that genre of text works: its structure and its limitations.
The Joking Computer specifically, and text generators in general, also manifest the nature of the data that they’re built upon. Take this joke (please):
What do you call a washing machine with a september?
An autumn-atic washer.
This joke shows the gaps that exist in the program’s data, and the unique way in which the program uses that data. First, the program doesn’t have a way to know that a washing machine isn’t a kind of thing that is likely to be “with” a “September” (or that September isn’t a noun likely to be used with an indefinite article). Second, the relationship between “September” and “autumn” depends on the eccentricity of WordNet, which claims that there is a meronymic relationship between the two concepts. The joke is constructed on the basis of the fact that September is a “part of” autumn—which certainly makes a kind of sense, but isn’t necessarily something that most people would intuitively agree with. The joke, as a consequence of (at least) these two factors, seems stilted and alien.
Then again, stilted or not, I happen to think this joke (“autumn-atic washer”!) is hilarious, and that the humor stems at least in part from the gaps in the data and way the program uses that data. Jokes, after all, are funny when they provide surprising juxtapositions or reconceptualizations of things in the world, and the program delivers those in abundance.
Poems succeed when they make use of similar juxtapositions and reconceptualizations. I think that this is why generative text programs are most effective when they are designed to generate text in these genres (humor and poetry). These programs succeed just because they don’t perfectly model the genre they set out to emulate.
In other genres, like conversation or narrative, surprising juxtapositions are less valued, or even specifically forbidden. I think that generative algorithms in those genres tend to be less successful for this very reason. But that’s a subject for another post.
(The Joking Computer via, more info)